How Do AI Art Generators Work?
AI-generated images come to life with just a text-based prompt in an AI art tool. Curious about the process behind the scenes? This article reveals it all.
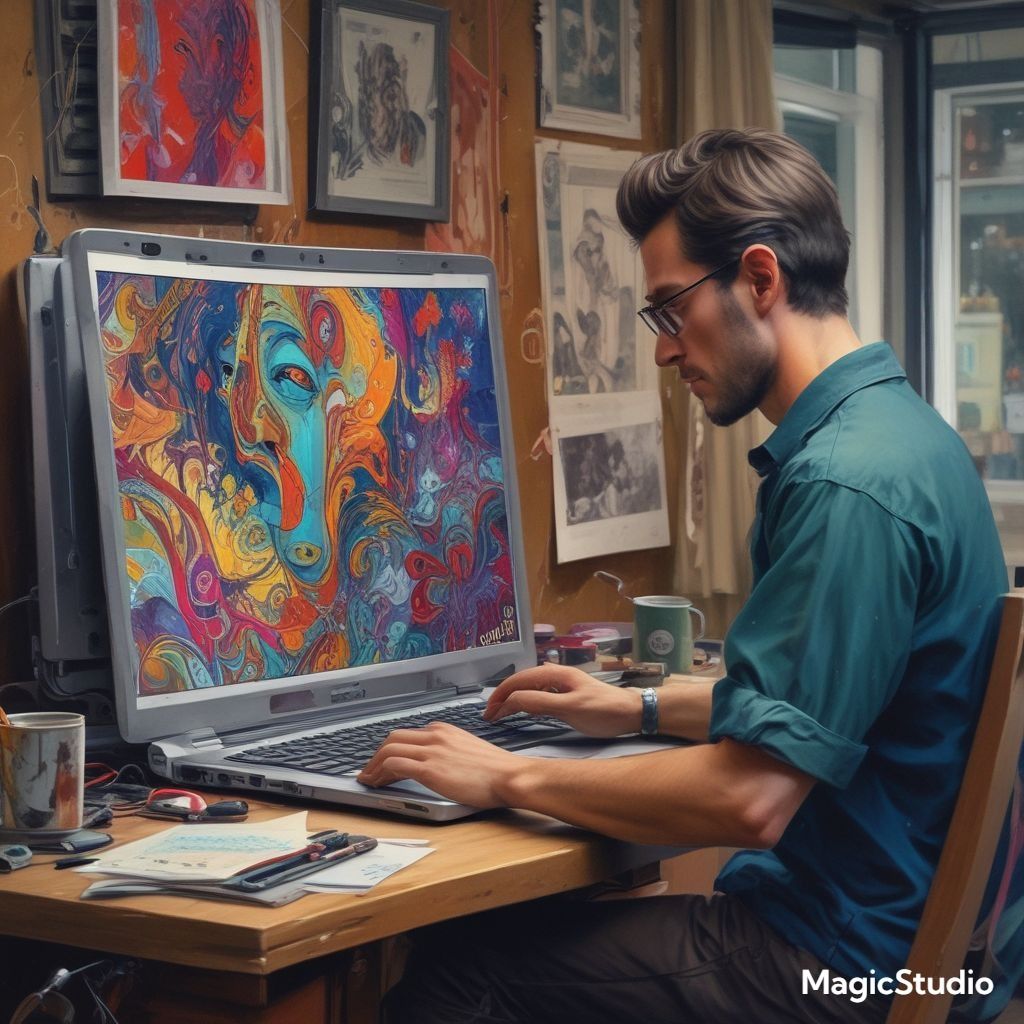
Are you one of those who are often surrounded by thoughts regarding AI mechanisms? And that too, especially when it comes to AI art generation. Isn't it just amazing how you can artify a random thought of yours by simply entering a suitable prompt in an AI art generating tool?
You might have loved it when you got the desired visual content that suited your work aesthetic. Getting images available was never so easy. It might appear as magic, but there is a robust technology that is making everything happen effortlessly before us.
You don't have to puzzle yourself anymore. It's time to say hello to the frequent question regarding the AI art generator's working mechanism that keeps poking you. Let's upgrade our AI art generation knowledge this time.
AI Art Generators
You might have heard of a variety of art generators and you might be acquainted with the functioning of some of these generators. DALL.E.2, Microsoft Designer, Magic Studio, and Canva; the list goes on.
Let us consider one of such amazing AI art generators, AI Art Generator by Magic Studio. As the name suggests, the outcome you get seems no less than magic. But this magic has a technical angle to it.
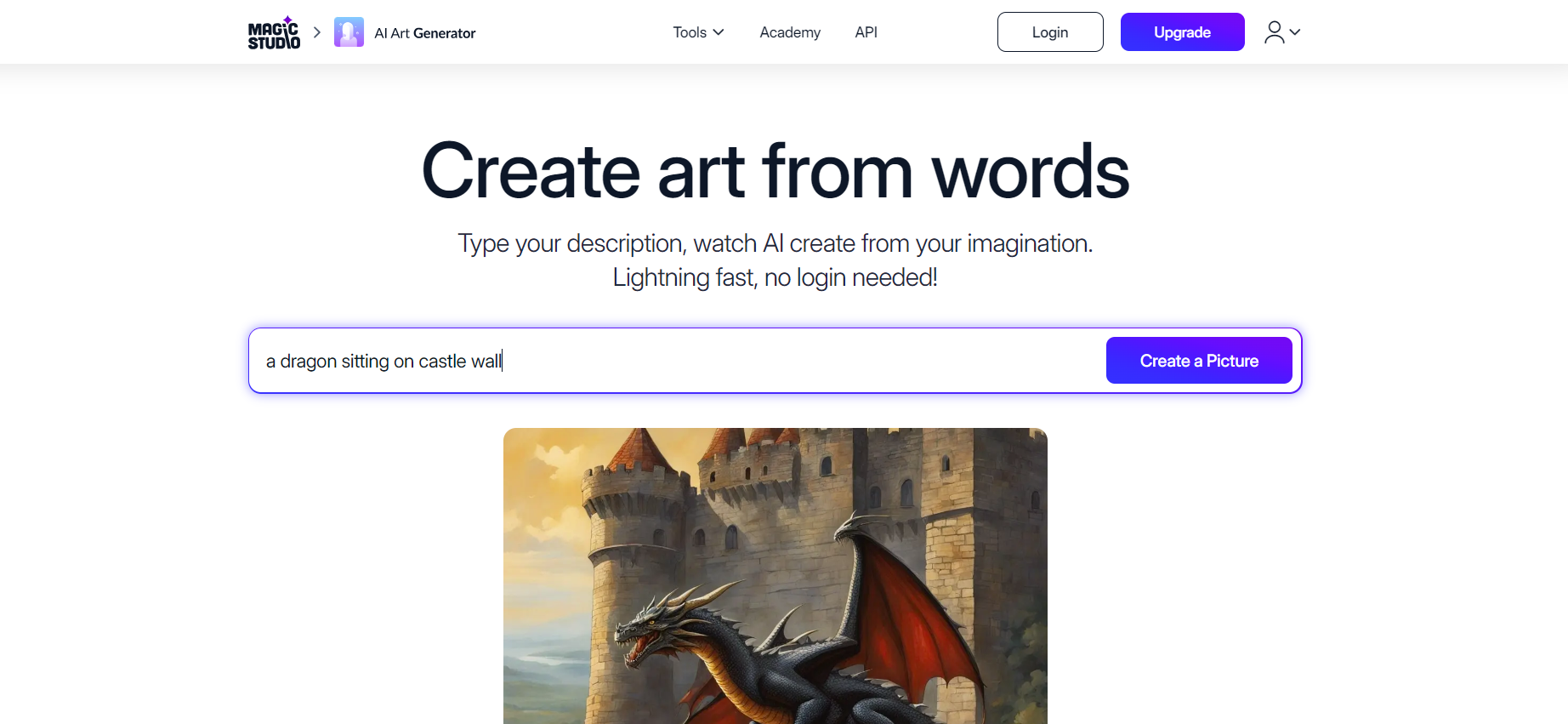
AI tools like Magic Studio are initially fed with large datasets. It consists of millions and millions of images and texts. A machine can't distinguish between two objects unless it is trained well. These tools are fed with datasets until they start recognising a particular object and its associated text.
For example, if you are entering the prompt “A standing white horse”, then the tool must be able to recognise the creature horse with the added attribute of being white. For this, the AI art generator is already saturated with datasets which will help it to analyze your prompt and display the desired outcome.
The ability of machines to analyze the prompt and decide the output is backed by technology, and this technology is based on a neural network. The neural network is basically an algorithm that helps the tool distinguish specific data from huge datasets. It is with the help of this algorithm that tools like Magic Studio can identify a horse from millions of datasets that are fed into the system. With the help of machine learning algorithms and neural networks, such tools can identify additional attributes.
Training AI Models for Art
When you talk about an apple, only a sensible and perceptive human brain can picture an apple. So, how can a machine portray an apple when it is instructed to? Does it even have such intelligence? Well, partially, it does, and that is why we call it “Artificial Intelligence”.
A machine can possess such intelligence only when it is trained well with several algorithms. When you enter a prompt in an AI art generator describing a mango tree, it uses the information it has learned about what a mango tree looks like. It must be able to distinguish a mango tree from all other trees that it has ever learned about.
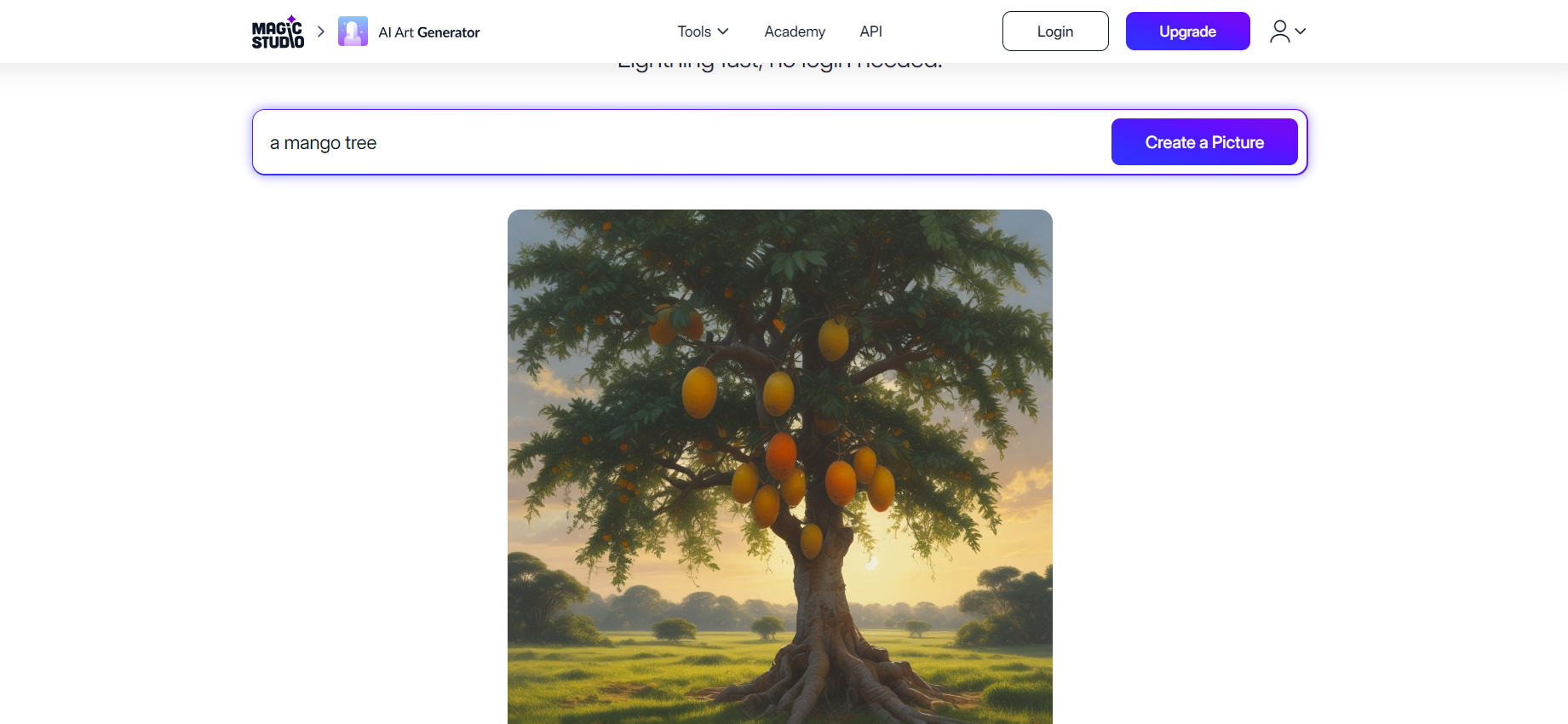
It then redefines the processing according to the additional attributes that you put in. The AI art generator that you are working on has massive image collections. It finds patterns that are similar to your prompt.
To deliver the desired results to you, an AI art generator must identify the prompt that you enter. For this, the AI art generating tools are trained with text-image pairs. The algorithm and information are set in such a manner that these tools are not confused with texts.
From Text Prompt to Image
The text that you provide is converted into an image through a process called Natural Language Processing. This process involves machine learning algorithms to interpret human language. This text-to-image generation uses deep learning models such as Generative Adversarial Networks (GAN) and Variational Autoencoders (VAE) to generate images from text.
The data sets that are initially fed into the AI are crucial for teaching the AI system to understand the relationship between text and visual content. The text that you enter as a prompt signals the system to identify the pattern among the datasets to create an image that best suits the prompt.
It must be noted that in text-to-image generation the quality of images generated depends widely on certain factors, like the complexity of text entered, the quality of training datasets, the architecture of AI models and the technique involved. Certain AI models like DALL.E, Midjourney and AI Art Generator by Magic Studio are acclaimed to generate quality images.
You can always refine your prompt and get better images. You must be very specific with the kind of image that you want. The AI art generator will process your text, and the image generated will entirely depend on your text.
So, be careful while entering the prompt.
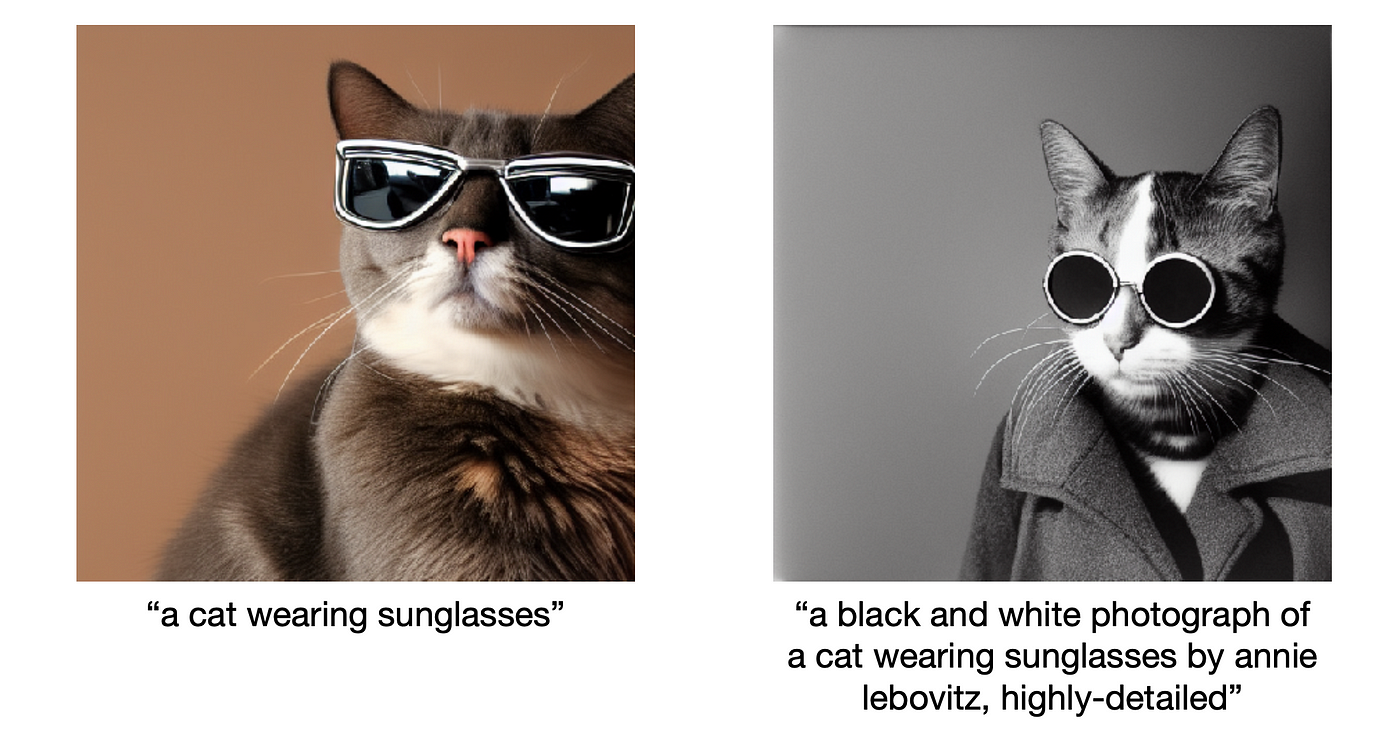
Technology Behind AI Art
Although each AI art generation tool has some unique features that make it different from others, the basic technology that is used in the AI art generation is more or less the same. Let us understand some basic generative AI models-
Generative Adversarial Networks (GANs)
GANs have two neural networks, trained on similar data, and they assist each other. The first network is responsible for generating the image while the second one is responsible for analyzing whether the image is newly created or just an original photo. For instance, if the first network creates an image of a butterfly, the second network will determine whether the image is newly created or simply acquired from the available datasets.
Variational Autoencoders (VAEs)
VAEs are made up of two neural networks working in tandem. Each of these networks is assigned a different job. The first network acts as an encoder, which takes the information. The second network acts as a decoder, which interprets the information and delivers the required image.
Diffusion Models
The diffusion model is a deep neural network that is capable of analyzing the structure of an image and redefining it for a better visual. It profoundly denoises the image to create a better version. It studies the information, follows the machine learning algorithms and creates a new image with variations. Diffusion models currently offer state-of-the-art performance in generative AI for images.
Perfection is a Myth: Limitations of AI Art Generation
The Myth of Perfection in AI Art
While AI art generation tools have made it possible for anyone to create digital artwork, the notion that these tools can produce flawless, perfect art is a myth. The images generated by AI may not always align perfectly with the user's intended vision or prompt.
Limitations of AI Art Generators
AI art generators rely on datasets and machine learning algorithms, which means they are limited by the information and biases present in their training data. They lack the human emotion, creativity, and intuition that can improve an artwork beyond the constraints of the input data.
As a result, the output of AI art generators may not always match the user's prompt or vision. Users may need to refine their prompts multiple times to get an output that is closer to their desired result.
Legal and Ethical Concerns
The rise of the AI art generation raises significant legal and ethical concerns. For instance, the ownership and copyright of AI-generated art are unclear, and there are ongoing debates about whether AI-generated art can be considered original or if it is simply a derivative work. Additionally, the potential for AI-generated art to be used for malicious purposes, such as deepfakes or propaganda, is a growing concern.
The Devaluation of Human-Made Art
The proliferation of AI-generated art on the internet has led to a blurring of the distinction between human-made and AI-generated artwork. This has resulted in the devaluation of human-made art, as it becomes increasingly difficult to differentiate between the two.
The Future of AI Art Generation
While AI art generation is a rapidly evolving field, it is important to recognize its limitations and not to overstate its capabilities. As we move further into the era of flourishing artificial intelligence, it is crucial to be cautious in our use and application of these tools and to maintain a balanced perspective on their strengths and weaknesses.
Recommendations for Responsible AI Art Generation
- Transparency: AI art generators should provide clear information about their processes and limitations to users.
- Ethical Considerations: The AI art generation should be used responsibly, avoiding the creation of harmful or misleading content.
- Copyright and Ownership: Clear guidelines on the ownership and copyright of AI-generated art are necessary to protect the rights of creators and users.
- Education and Awareness: Educating users about the limitations and potential risks of AI art generation is essential to promote responsible use.
Final Words
AI art generation is a powerful tool that has the potential to change the way we create and interact with art completely. However, it is crucial to recognise its limitations and the legal and ethical concerns that arise from its use. By being aware of these limitations and taking steps to promote responsible use, AI art generation can be used to benefit both creators and society as a whole.